Mitsuo Kawato
ATR Computational Neuroscience Labs, Japan
Title: Towards Manipulative Neuroscience based on Brain-Network-Interface
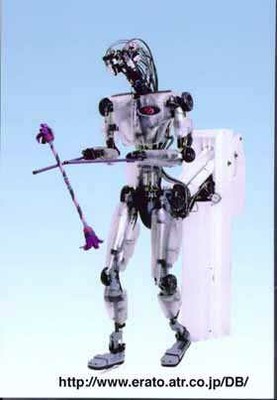
Abstract: The cerebellar internal model theory postulates that the cerebellar cortex acquires many internal models of controlled objects, dynamical processes in the external world dependent on long-term depression (LTD) of Purkinje cells. It predicts that the climbing fiber inputs to Purkinje cells carry the feedback motor command and can supervise learning of inverse dynamics models. Many experimental supports were obtained from the ventral paraflocculus of the cerebellum during monkey control of ocular following responses. fMRI studies mapped forward and inverse models of manipulated objects and tools in the cerebellar cortex. Kinetic models of LTD [1,2] suggest a cascade of excitable and bistable dynamical processes, which may resolve plasticity-stability dilemma at single spine level. That is, even a single pulse of climbing fiber input combined with an early train of several parallel fiber pulses can induce Ca2+ induced Ca2+ release via IP3 receptors on ER. The MAPK positive feedback loop leaky integrates resulting large Ca2+ elevation and if it crosses the threshold then the state moves to the depressed equilibrium. These models explain diverse LTD experiments and clearly demonstrate that LTD is a supervised learning rule, and not anti-Hebbian as erroneously characterized. The MAPK positive feedback loop model [1] was recently supported by a Ca2+ photo-uncaging and imaging experiment [3] that supports LTD all-or-none character. In [3], the most important information within the system, Ca2+ can be measured and manipulated directly, thus the theory was quite rigorously proved. At the system level, the cerebellar internal models for arm movements are still debated and we need to develop a method to directly manipulate information.
In our "Understanding brain by Creating Brain"-approach, high-performance humanoid robots have been developed, and sensory-motor coordination problems were investigated. We explored several computational theories such as MOSAIC, imitation learning, biologically motivated robot biped locomotion, modular and hierarchical reinforcement learning models. Our paradigms include computational-model based imaging and non-invasive decoding of neural representations. However, we got frustrated that most experiments can show just temporal correlation between data and theory but not causality, unlike the above spine-level LTD story. We hope that manipulative neuroscience based on real time feedback of decoded neural information could be a resolution to this difficulty. Hierarchical Bayesian approach to combine fMRI and MEG, or NIRS and EEG, or neural decoding with machine learning techniques, and real-time control of robots with decoded information are expected to be key technological elements. Demonstrations of real-time robot hand control by decoded information from fMRI (http://www.atr.jp/html/topics/press_060526_e.html) in collaboration with Honda, control of a humanoid robot locomotion-like movements from monkey brain in collaboration with Miguel Nicolelis [4], prediction of wrist joint angular acceleration from the primary motor cortex currents estimated by hierarchical Bayesian MEG, or estimation of visual target velocity from MST currents, etc could be technical bases for this new approach.
[1] Kuroda S, Schweighofer N, Kawato M: Exploration of signal transduction pathways in cerebellar long-term depression by kinetic simulation. Journal of Neuroscience, 21: 5693-5702, 2001.
[2] Doi T, Kuroda S, Michikawa T, Kawato M: Insoitol, 1, 4, 5-trisphosphate-dependent Ca2+ threshold dynamics detect spike timing in cerebellar Purkinje Cells. Journal of Neuroscience, 25: 950-961, 2005.
[3] Tanaka K, Khiroug L, Santamaria F, Doi T, Ogasawara H, Ellis-Davies G, Kawato M, Augustine GJ: Ca2+ requirements for cerebellar long-term synaptic depression: role for a postsynaptic leaky integrator, Neuron, 54: 787-800, 2007.
[4] Kawato M: Brain controlled robots. HFSP Journal, doi:10.2976/1.2931144 (2008)
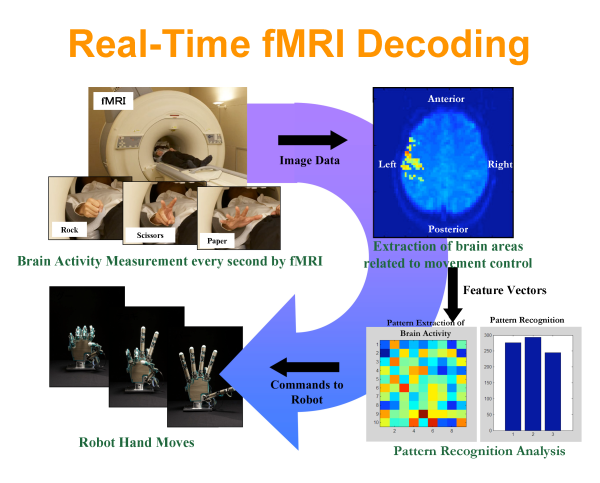
Bio-sketch: Prof. Kawato received the B.S. degree in physics from Tokyo University in 1976 and the M.E. and Ph. D. degrees in biophysical engineering from Osaka University in 1978 and 1981, respectively. From 1981 to 1988, he was a faculty member and lecturer at Osaka University. Professor Kawato has served as a director of ATR computational Neuroscience Laboratories and a research supervisor of JST, ICORP Computational Brain Project. He is now concurrently working as a visiting professor at Kanazawa Institute of Technology, Nara Institute of Science and Technology, Osaka University, the National Institute for Physiological Sciences and Kyoto Prefectural University of Medicine. He has been appointed Toyama Prefectural University as a Specially Appointed Visiting Professor. He was awarded the Yonezawa founder's medal memorial special award of The Institute of Electronics, Information and Communication Engineers, in 1991, the outstanding research award of the International Neural Network Society in 1992, the Osaka Science Prize in 1993, the 10th Tsukahara Naka-akira Memorial Award in 1996, the Tokizane Toshihiko Memorial Award in 2001, IEICE fellow in 2004, the Chunichi Cultural Award and the Shida Rinzaburo Award in 2005, the Asahi Prize in 2007. He is a governing board member of the Japanese Society of Neuroscience and a Member of American Physiologica Society. He is currently serving as an Editor of HFSP Journal.
For the last 15 years he has been working in computational neuroscience and neural network modeling. He published about 200 papers, reviews and books. Research topics include simulation study of dendritic spines, feedback-error-learning model and its applications to industrial robot manipulators, movement trajectory formation, bi-directional theory for interactions between cortical areas, cerebellar internal models, and teaching by demonstration for robots.
Professor Kawato’s work focuses on constructing a brain in order to understand the brain, through building a brain to the extent that we can build a brain. More concretely, he has been investigating the information processing of the brain with the long-term goal that machines, either computer programs or robots, could solve the same computational problems as those that the human brain solves, while using essentially the same principles. With these general approaches, he has greatly contributed in elucidating visual information processing, optimal control principles for arm trajectory planning, internal models in the cerebellum, teaching by demonstration for robots, human interfaces based on electoromyogram, and applications in rehabilitation medicine. Recently, he proposes a new experimental paradigm; manipulative neuroscience.